💻 All Article
[CV] Object Detection, COCO- How to train Detectron2 with Custom COCO Datasets
Instance Segmentation Examplehttps://github.com/wkentaro/labelme/tree/main/examples/instance_segmentationHow to train Detectron2 with Custom COCO Datasetsmulti classfromdetectron2.data.datasetsimport register_coco_instancesregister_coco_instances("fruits_nuts", {}, "./data/trainval.json", "./data/images")https://www.dlology.com/blog/how-to-train-detectron2-with-custom-coco-datasets/..
[CV] Object Detection, COCO dataset 만들기
가상환경 만들기https://www.dlology.com/blog/how-to-create-custom-coco-data-set-for-instance-segmentation/ How to create custom COCO data set for instance segmentation | DLologyPosted by: Chengwei 3 years ago (Comments) In this post, I will show you how simple it is to create your custom COCO dataset and train an instance segmentation model quick for free with Google Colab's GPU. If you just wan..
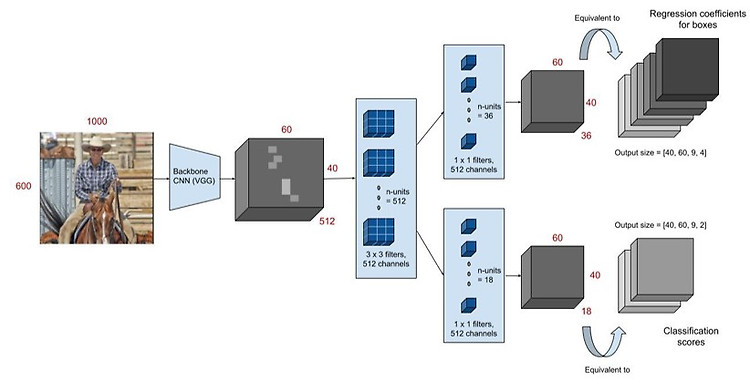
[논문 리뷰] Faster R-CNN: Towards Real-Time Object Detection with Region Proposal Networks,Shaoqing Ren et.al., NIPS 2015
Fast R-CNN notable drawback Fast rcnn은 Selective Search가 독립적으로 수행되었기 때문에 bottleneck이 발생하였습니다.(region proposal은 CPU연산이고 region-based CNN은 GPU 연산) 그래서 detection 네트워크의 성능을 아무리 개선시켜 봤자 Selective Search, region proposals의 시간은 그대로 추가가 되기 때문에 속도를 개선시키지 못한다는 단점이 있었습니다. 실시간 추론을 목표로 제안 했기 때문에 매우 빠른 추론이 가능하다 1. Faster R-CNN: RPN + Fast R-CNN 그래서 faster rcnn은 Cpu에서 진행되는 Selective search의 방식이 아닌 Region propo..
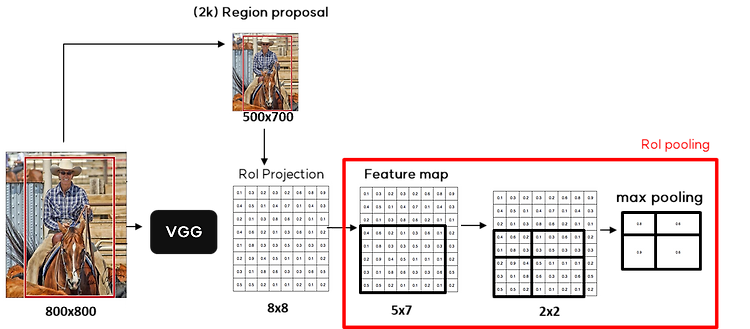
[논문 리뷰] Fast R-CNN: Ross Girshick, ICCV 2015
R-CNN notable drawback 1.Training is a multi-stage pipeline 2.The image was forcibly warped to a size of 224x224 3.No back propagation 4.Training is expensive in space and time(disk, 2.5 GPU-days) 2000개의 Image Proposal 후보를 모두 CNN 모델에 집어 넣기 때문에, training, testing 시간이 매우 오래 걸림. AlexNet을 그대로 사용하기 위해 Image를 224x224 크기로 강제로 warping 시켰기 때문에 이미지 변형으로 인한 성능 손실이 존재 뒷 부분에서 수행한 Computation을 Share하지 않는다. (N..